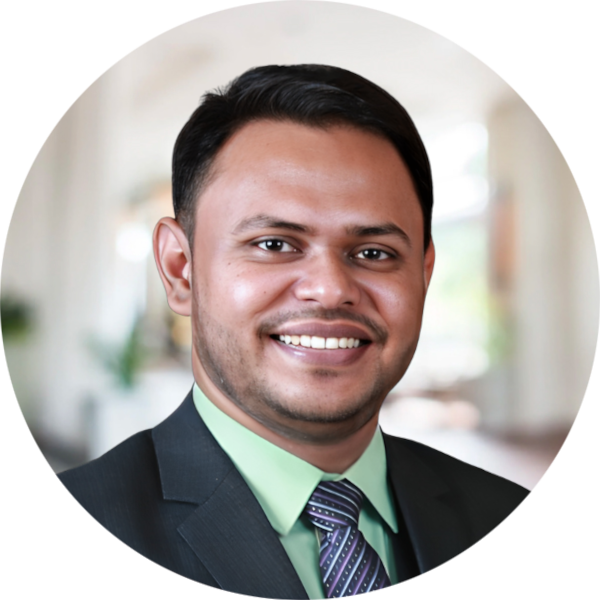
Hello World!!
This is Imran, born and raised in the city of mosques, Dhaka, Bangladesh. I am a passionate programmer, Data Science/Machine Learning professional and a researcher. I help companies make impactful data-driven decisions through utilizing and productionalizing AI and Data Science technologies. I’m a goal-oriented seasoned Data Science and Machine Learning professional with deep affection for data and proven expertise in developing and deploying end-to-end, highly scalable ML models and services. Predicting unknowns, discovering patterns, and revealing useful insights from data excites me the most. I’m dynamic in personality and a rapid learner who is always desperate for knowledge and wisdom.
Technical Skills
Publications [Citations: 314, h-index: 10, i10-index: 10]
Abdullah Al Imran, Md Farhan Ishmam. "FourierKAN outperforms MLP on Text Classification Head Fine-tuning." arXiv preprint arXiv:2408.08803 (2024). https://doi.org/10.48550/arXiv.2408.08803v2
Abstract: In resource constraint settings, adaptation to downstream classification tasks involves fine-tuning the final layer of a classifier (i.e. classification head) while keeping rest of the model weights frozen. Multi-Layer Perceptron (MLP) heads fine-tuned with pre-trained transformer backbones have long been the de facto standard for text classification head fine-tuning. However, the fixed non-linearity of MLPs often struggles to fully capture the nuances of contextual embeddings produced by pre-trained models, while also being computationally expensive. In our work, we investigate the efficacy of KAN and its variant, Fourier KAN (FR-KAN), as alternative text classification heads. Our experiments reveal that FR-KAN significantly outperforms MLPs with an average improvement of 10% in accuracy and 11% in F1-score across seven pre-trained transformer models and four text classification tasks. Beyond performance gains, FR-KAN is more computationally efficient and trains faster with fewer parameters. These results underscore the potential of FR-KAN to serve as a lightweight classification head, with broader implications for advancing other Natural Language Processing (NLP) tasks.
Abdullah Al Imran, Md Sakib Hossain Shovon, and M. F. Mridha. "BaitBuster-Bangla: A Comprehensive Dataset for Clickbait Detection in Bangla with Multi-Feature and Multi-Modal Analysis." In Data in Brief (2024): 110239. https://doi.org/10.1016/j.dib.2024.110239
Abstract: This study presents a large multi-modal Bangla YouTube clickbait dataset consisting of 253,070 data points collected through an automated process using the YouTube API and Python web automation frameworks. The dataset contains 18 diverse features categorized into metadata, primary content, engagement statistics, and labels for individual videos from 58 Bangla YouTube channels. A rigorous preprocessing step has been applied to denoise, deduplicate, and remove bias from the features, ensuring unbiased and reliable analysis. As the largest and most robust clickbait corpus in Bangla to date, this dataset provides significant value for natural language processing and data science researchers seeking to advance modeling of clickbait phenomena in low-resource languages. Its multi-modal nature allows for comprehensive analyses of clickbait across content, user interactions, and linguistic dimensions to develop more sophisticated detection methods with cross-linguistic applications.
Abdullah Al Imran, and and Kasun S. Perera. "Large-scale Probabilistic Forecasting of Consumer Engagement of CPG Products using Heterogeneous Web Data." In Procedia Computer Science 237 (2024): 426-436. https://doi.org/10.1016/j.procs.2024.05.124
Abstract: Consumer Packaged Goods (CPG) play a pivotal role in customer-centric industries. Understanding the distinctive features of such products and how customers engage with them is essential for CPG manufacturers to create customer-winning products. In this study, we explore an innovative data-centric approach to forecast customer engagement for CPG products by monitoring their digital evolution, particularly in the Snacks category in the USA. Traditional methods for consumer analysis such as surveys, focus groups are time consuming, costly and ineffective with limited scope. They also lack a forecasting component, making it difficult for CPG companies to make forward-looking decisions. However, with the emergence of big data, we can leverage user generated public data from social media, web search, e-commerce platforms to estimate consumer engagement and make long-term forecasts. To achieve this, we propose a systematic approach to accumulate and prepare large datasets from heterogeneous web sources for CPG products. We then use state-of-the-art deep learning based time series forecasting models to efficiently train and predict consumer engagement for the next 12 months, benchmarking their computational efficiency and forecasting performance. Our findings indicate that the DeepAR model outperforms all other models, with the lowest NRMSE (=0.378), RMSE (=14.848) MSE (=220.457), MASE (=0.871), and sMAPE (=0.306) values. Furthermore, we demonstrate methods for computing single forecasting points and prediction intervals using the forecasted sample distribution from the probabilistic models. The proposed approach will provide CPG businesses with valuable insights to make informed decisions about product development, marketing strategies, and supply chain management.
Amin, Md Nur, Abdullah Al Imran, and Alexander Jesser. "Adversarial Debiasing using Regularized Direct Entropy Minimization in Wasserstein GAN with Gradient Penalty." In 2023 26th International Conference on Computer and Information Technology (ICCIT), pp. 1-6. IEEE, 2023. https://doi.org/10.1109/ICCIT60459.2023.10441224
Abstract: The promise of machine learning algorithms are overshadowed by the ethical challenges they present, especially when biases in data lead to discriminatory outcomes based on gender or other sensitive attributes. This paper introduces an innovative methodology to address gender bias in machine learning models, particularly when processing textual data. By harnessing the power of adversarial training with the Wasserstein GAN enhanced by gradient penalty (WGAN-GP), our approach strives to minimize gender-specific information in the model’s latent representations. Central to our methodology is the integration of a Direct Entropy Minimization (DEM) regularizer, which further ensures that these representations are devoid of gender biases. The dual objective, achieved through our primary classifier and its adversarial counterpart, not only maintains high classification accuracy but also substantially eliminates gender bias.
Amin, Md Nur, Abdullah Al Imran, Fatih S. Bayram, Lea Hübner, and Alexander Jesser. "Breaking Bias: Alpha Weighted Loss in Multi-objective Learning Taming Gender Stereotypes." In World Conference on Information Systems for Business Management, pp. 233-246. Singapore: Springer Nature Singapore, 2023. https://doi.org/10.1007/978-981-99-8346-9_20
Abstract: Navigating the uncertainties of job classification and gender bias, this paper presents multi-objective learning approach using BERT-based model that concurrently handles maximizing accuracy and mitigating gender bias. Main contribution of this study is making use of a loss function with a trade-off parameter, acknowledging no definitive ‘optimal’ solution is presumed. Eliminate unwanted bias or refrain systems from reinforcing bias seeking to unjust impact on people to sensitive characteristics is a critical consideration. This research underscores the pivotal role of decision-making under uncertainty in AI, setting a precedent for more conscious, bias-aware AI system design.
Akter, Shamima, Manik Ahmed, Abdullah Al Imran, Ahsan Habib, Rakib Ul Haque, Md Sohanur Rahman, Md Rakibul Hasan, and Samira Mahjabeen. "CKD.Net: A novel deep learning hybrid model for effective, real-time, automated screening tool towards prediction of multi stages of CKD along with eGFR and creatinine." In Expert Systems with Applications 223 (2023): 119851. https://doi.org/10.1016/j.eswa.2023.119851
Abstract: Clinical tests have long been considered appropriate in diagnosing chronic kidney disease (CKD) because of their noninvasiveness, simplicity, and cost. Timely detection and management of CKD are the most effective methods to address the expanding global burden induced by CKD. We adopted an S-MTL (Supervised Multi-task Learning) approach and combined SimpleRNN (Simple Recurrent Neural Network) and MLP (Multi-Layer Perception) to develop a hybrid model-CKD.Net to predict five CKD stages. This hybrid neural network architecture was trained on massive clinical datasets with heterogeneous 27 features to predict kidney function. We employed various data augmentation strategies to balance the five CKD stage datasets and meticulously utilized the hyperparameter to minimize the loss and validation loss to reduce overfitting and hence increase model generalization. Performance comparisons of CKD.Net were evaluated using Accuracy, Precision, Recall, and F1-score while comparing the performance with that of generic SimpleRNN and MLP models. CKD.Net demonstrated superior classification accuracy ranging from 99.2 to 99.8 percent in predicting the five classes. Furthermore, CKD.Net was utilized to predict eGFR (estimated glomerular filtration rate) and creatinine by evaluating the confidence level using Pearson correlation values. Subsequently, key risk factors of CKD were identified, and their clinical significance was discussed. CKD.Net web application was developed to automate the prediction of CKD disease. To the best of our knowledge, the CKD.Net model is the first essential step toward predicting multi-stages of kidney disease as an effective, real-time, automated screening tool. CKD.Net allows noninvasive measurement of kidney function, which is a crucial objective of artificial intelligence powered by functional automation in clinical practice.
Dolly Agarwal, Abdullah Al Imran, and Kasun S Perera. "FOODIE: A Data-centric Sifting Framework for Social Media Analytics." In 35th International Conference on Software Engineering and Knowledge Engineering, 2023. https://doi.org/10.18293/SEKE23-175
Abstract: There has been a great deal of research conducted in the past on utilizing social media analytics to derive consumer insights and understand their behaviors. However, when such studies are applied to real-world data in an industrial use-case, the results are often found to be incorrect and erroneous. This is a major barrier for companies that provide social media analytics-based solutions to customer-centric industries such as Food and Beverage (FnB). One of the key causes of this barrier is the failure to appropriately process and curate raw social data prior to analytics. In this study, we discuss the challenges we encountered when dealing with social data throughout our industrial experience and propose a standard solution - FOODIE. This is a framework specifically designed for the FnB industry to process social conversation data accurately and in a standard manner prior to perform various downstream tasks on it. The three stages of this paradigm are preparation, sifting, and evaluation. Through this framework, we have reduced the data to error ratio from 8.76% to 0.01% which is quite significant given the volume of the data. While this framework is designed for the FnB space, it can be customized to suit the needs of various other industries.
Wahid, Zaman, and Abdullah Al Imran. "Multi-feature Transformer for Multiclass Cyberbullying Detection in Bangla." In IFIP International Conference on Artificial Intelligence Applications and Innovations, pp. 439-451. Cham: Springer Nature Switzerland, 2023. https://doi.org/10.1007/978-3-031-34111-3_37
Abstract: Cyberbullying detection is a global issue that must be addressed to improve the cyberspace for millions of online users, services, and organizations. Online harassment of the general public and celebrities is now commonplace on social media, particularly in Bangladesh. In this paper, we present a novel multi-feature transformer followed by a deep neural network for multiple-dimensional cyberbullying detection. Using online Bangla textual data, we introduce the user’s social profile, the lexical features, the contextual embedding, and the semantic similarities among word associations in Bangla in order to develop an effective and robust cyberbullying detection system. Our proposed method can detect cyberbullying in Bangla with a 98% detection accuracy for threats and a 90% detection accuracy for sarcastic comments. The aggregate accuracy of all six multiclass labels is 86.3%. In addition, the experimental results find that the proposed technique outperforms the state-of-the-art methods for detecting cyberbully in Bangla.
Wahid, Zaman, Abdullah Al Imran, and Md Rifatul Islam Rifat. "BNnetXtreme: An Enhanced Methodology for Bangla Fake News Detection Online." In International Conference on Computational Data and Social Networks, pp. 157-166. Cham: Springer Nature Switzerland, 2022. https://doi.org/10.1007/978-3-031-26303-3_14
Abstract: In the last couple of years, the government, and the public have shown a lot of interest in fake news on Bangladesh’s fast-growing online news sites, as there have been significant events in various cities due to unjustifiable rumors. But the overall progress in study and innovation in the detection of Bangla fake and misleading news is still not adequate in light of the prospects for policymakers in Bangladesh. In this study, an enhanced methodology named BNnetXtreme is proposed for Bangla fake news detection. Applying both embedding based (i.e. word2vec, Glove, fastText) and transformer-based (i.e. BERT) models, we demonstrate that the proposed BNnetXtreme achieves promising performance in detection of Bangla fake news online. After a further comparative analysis, it is also discovered that BNnetXtreme performed superior to BNnet-one of the state-of-the-art architectures for Bangla fake news detection introduced previously. The BNnetXtreme especially BERT Bangla base model performed with an accuracy score of 91% and an AUC score of 98%. Our proposed BNnetXtreme has been successful in improving the performance by an increase of 1.1% in accuracy score, 5.6% in precision, 1.1% in F1 score, and about 9% in AUC score.
Rifat, Md Rifatul Islam, Md Nur Amin, Mahmud Hasan Munna, and Abdullah Al Imran. "An End-to-end Machine Learning System for Mitigating Checkout Abandonment in E-Commerce." 2022 17th Conference on Computer Science and Intelligence Systems (FedCSIS). IEEE, 2022. https://doi.org/10.15439/2022F167
Abstract: Electronic Commerce (E-Commerce) has become one of the most significant consumer-facing tech industries in recent years. This industry has considerably enhanced people’s lives by allowing them to shop online from the comfort of their own homes. Despite the fact that many people are accustomed to online shopping, e-commerce merchants are facing a significant problem, a high percentage of checkout abandonment. In this study, we have proposed an end-to-end Machine Learning (ML) system that will assist the merchant to minimize the rate of checkout abandonment with proper decision making and strategy. As a part of the system, we developed a robust ML model that predicts if someone will checkout the products added to the cart based on the customer’s activity. Our system also provides the merchants with the opportunity to explore the underlying reasons for each single prediction output. This will indisputably help the online merchants in business growth and effective stock management.
Abdullah Al Imran, Zaman Wahid, Alpana Akhi Prova, and Md Hannan. "Harnessing the meteorological effect for predicting the retail price of rice in Bangladesh." International Journal of Business Intelligence and Data Mining 20, no. 4 (2022): 440-455. https://doi.org/10.1504/IJBIDM.2022.123215
Abstract: Bangladesh has seen an absurd, steeper prize-hike for the last couple of years in one of the most consumed foods taken by millions of people every single day: rice. The impact of this phenomenon, however, is indispensably critical, especially to the one striving for daily meals. Thus, understanding the latent facts is vital to policymakers for better strategic measures and decision-making. In this paper, we have applied five different machine learning algorithms to predict the retail price of rice, find out the top-most factors responsible for the price hike, and determine the best model that produces higher prediction results. Leveraging six evaluation metrics, we found that random forest produces the best result with an explain variance score of 0.87 and an R2 score of 0.86 whereas gradient boosting produces the least, meanwhile discovering that average wind speed is the topmost reason for rice price hike in retail markets.
Rifat, Md Rifatul Islam, and Abdullah Al Imran. "Incorporating Transformer Models for Sentiment Analysis and News Classification in Khmer." International Conference on Computational Data and Social Networks. Springer, Cham, 2021. https://doi.org/10.1007/978-3-030-91434-9_10
Abstract: In recent years, natural language modeling has achieved a major breakthrough with its sophisticated theoretical and technical advancements. Leveraging the power of deep learning, transformer models have created a disrupting impact in the domain of natural language processing. However, the benefits of such advancements are still inscribed between few highly resourced languages such as English, German, and French. Low-resourced language such as Khmer is still deprived of utilizing these advancements due to lack of technical support for this language. In this study, our objective is to apply the state-of-the-art language models within two empirical use cases such as Sentiment Analysis and News Classification in the Khmer language. To perform the classification tasks, we have employed FastText and BERT for extracting word embeddings and carried out three different type of experiments such as FastText, BERT feature-based, and BERT fine-tuning-based. A large text corpus including over 100,000 news articles has been used for pre-training the transformer model, BERT. The outcome of our experiment shows that in both of the use cases, a pre-trained and fine-tuned BERT model produces the outperforming results.
Abdullah Al Imran, and Md Nur Amin. "Deep Bangla Authorship Attribution Using Transformer Models." International Conference on Computational Data and Social Networks. Springer, Cham, 2021. https://doi.org/10.1007/978-3-030-91434-9_11.
Abstract: Authorship attribution is one of the renowned problems in the domain of Natural Language Processing (NLP). Leveraging the state-of-the-art (SOTA) techniques of NLP such as transformer models, this problem domain has achieved a considerable advancement. However, this progress is unfortunately only bound to the well-resourced languages like English, French, and German. Under-resourced language like Bangla is yet to leverage such SOTA techniques to make a breakthrough in this domain. In this study, we address this research gap and aim to contribute to the Bangla authorship attribution problem by building highly accurate models using several SOTA variants of transformer models like mBERT, bnBERT, bnElectra, and bnRoBERTa. Using the pre-trained weights of these models we have performed fine-tuning and tackled the task of authorship attribution of 16 prominent Bangla writers. Outcomes show that our bnBERT model can classify the authors with superior accuracy of 98% and also outperform all the existing models available in the literature.
Abdullah Al Imran, Md Shamsur Rahim, and Tanvir Ahmed. "Mining the Productivity Data of the Garment Industry." International Journal of Business Intelligence and Data Mining. Inderscience Publishers, 2021. https://doi.org/10.1504/ijbidm.2021.118183.
Abstract: The garment industry is one of the key examples of the industrial globalisation of this modern era. It is a highly labour-intensive industry with lots of manual processes. Satisfying the huge global demand for garment products is mostly dependent on the production and delivery performance of the employees in the garment manufacturing companies. So, it is highly desirable among the decision makers in the garments industry to track, analyse and predict the productivity performance of the working teams in their factories. This study explores the application of state-of-the-art data mining techniques for analysing industrial data, revealing meaningful insights and predicting the productivity performance of the working teams in a garment company. As part of our exploration, we have applied eight different data mining techniques with six evaluation metrics. Our experimental results show that the tree ensemble model and gradient boosted tree model are the best performing models in the application scenario.
Abdullah Al Imran, and Md Nur Amin. “Loan Charge-Off Prediction Including Model Explanation for Supporting Business Decisions.” Advances in Intelligent Systems and Computing. Springer International Publishing, 2021. https://doi.org/10.1007/978-3-030-71187-0_119.
Abstract: The rapid growth of taking loans and digitizing the financial sector is increasing the rate of loan charge-offs as well as the volume of data that represents customer behavior. Nowadays, Machine Learning (ML) technology is helping financial institutions utilize this huge amount of data and build some black-box prediction models for predicting loan charge-offs with decent accuracy. Yet, the amount of risk involved in such financial decisions is very high and should not be taken only based on an opaque decision of a black-box model. In this study, we propose a system for building accurate models using interpretable state-of-the-art (SOTA) ML algorithms as well as utilizing the Explainable AI (XAI) techniques to explain individual instances for supporting business decisions.
Abdullah Al Imran, Zaman Wahid, and Tanvir Ahmed. “BNnet: A Deep Neural Network for the Identification of Satire and Fake Bangla News.” Computational Data and Social Networks. Springer International Publishing, 2020. https://doi.org/10.1007/978-3-030-66046-8_38.
Abstract: Misleading and fake news in rapidly increasing online news portals in Bangladesh has become a major concern to both the government and public lately, as a substantial amount of incidents have taken place in different cities due to unwarranted rumors over the last couple of years. However, the overall progress of research and innovation in detecting fake and satire Bangla news is yet unsatisfactory considering the prospects it would bring to the decision-makers of Bangladesh. In this study, we have amalgamated both fake and real Bangla news from quite a pool of online news portals and applied a total of seven prominent machine learning algorithms to identify real and fake Bangla news, proposing a Deep Neural Network (DNN) architecture. Using a total of five evaluation metrics: Accuracy, Precision, Recall, F1 score, and AUC, we have discovered that DNN model yields the best result with an accuracy and AUC score of 0.90 respectively while Decision Tree performs the worst.
Parves, Abdul Bari, Abdullah Al Imran, and Md. Riazur Rahman. “Incorporating Supervised Learning Algorithms with NLP Techniques to Classify Bengali Language Forms.” Proceedings of the International Conference on Computing Advancements. ACM, January 10, 2020. https://doi.org/10.1145/3377049.3377110.
Abstract: Every language has its own root, form, and grammar, and so does Bengali. Bengali language has two core forms: "Sadhu-bhasha" and "Cholito-bhasha" which have been widely used from regular communication to literary publications. At present, Sadhu-bhasha can be only found in old books and literary publications, whereas Cholito-bhasha is mostly used everywhere. However, so many Bengali linguists are still researching on these two forms to preserve its root, understand and develop Bengali, and also extract knowledge from the historical publications which were mainly written in Sadhu-bhasha. Unfortunately, till now they do not have any digital tool that can assist their research by automatically identifying these core forms of Bengali from the large archive of Bengali literature. This study aims to build such an automatic intelligent system that can accurately identify these two language forms by harnessing the power of Natural Language Processing (NLP). In this study, we have applied advanced NLP techniques and six Supervised learning algorithms to classify "Sadhu-bhasha" and "Cholito-bhasha" from text corpora. Results of this study show that all the six models yielded very promising results, however, the Multinomial Naive Bayes outperformed all the models with 99.5% accuracy, 99.0% precision, 100% recall, 0.995 AUC score and, 0.995 F1 score. Additionally, this study also performs qualitative analysis using t-SNE algorithm to visualize the difference between Sadhu-bhasha and Cholito-bhasha.
Abdullah Al Imran, and Md Nur Amin. “Predicting the Return of Orders in the E-Tail Industry Accompanying with Model Interpretation.” Procedia Computer Science. Elsevier BV, 2020. https://doi.org/10.1016/j.procs.2020.09.113.
Abstract: Electronic Retailing (E-tailing) is one of the most impactful technology trends of recent times. This industry has dramatically enhanced the quality of human lives allowing people to shop online while having the comfort of their homes. In developing countries like Bangladesh, this industry is still rising and creating a significant economic impact. However, there exist a lot of challenges such as the return of orders that affects the growth of an E-tailer and causes revenue losses. This study addresses this most common business challenge in the E-tail industry and performs predictive modeling using 4 different state-of-the-art data mining techniques to help the industry smoothen its curve of growth. Along with predictive modeling, this study also aims to find out the most important features that influence the return of orders.
Abdullah Al Imran, Md. Rifatul Islam Rifat, and Rafeed Mohammad. “Enhancing the Classification Performance of Lower Back Pain Symptoms Using Genetic Algorithm-Based Feature Selection.” Proceedings of International Joint Conference on Computational Intelligence. Springer Singapore, July 4, 2019. https://doi.org/10.1007/978-981-13-7564-4_39.
Abstract: Lower Back Pain (LBP) is one of the leading causes of disability around the world that affects several important parts of the human body such as the muscles, nerves, and bones of the back. The early diagnosis and proper treatment can only prevent acute LBP from infecting into chronic LBP. The aim of this study is to enhance the classification performance of LBP by identifying the most relevant feature subset from a broader feature space of an LBP dataset. To serve the aim, we have proposed a Genetic Algorithm (GA)-based feature selection approach that has been proved to significantly improve the classification performance of LBP. For the purpose of classification, we have used seven different classification algorithms, namely Logistic Regression, Ridge Regression, Gaussian Naive Bayes, Random Forest, Decision Tree, k-Nearest Neighbors (KNN), and Support Vector Machine (SVM). After applying our proposed GA-based feature selection approach along with the base classifiers, we have obtained a significant average increment in accuracy, precision, recall, f1-score, and AUC score by 3.1%, 0.64%, 4.37%, 2.64%, and 3.83% respectively. The k-Nearest Neighbors outperforms the other models with the highest accuracy (=85.2%), precision (=89.9%), and f1 score (=88.9%).
Islam Rifat, Md Rifatul, Abdullah Al Imran, and A. S. M. Badrudduza. “Educational Performance Analytics of Undergraduate Business Students.” International Journal of Modern Education and Computer Science. MECS Publisher, July 8, 2019. https://doi.org/10.5815/ijmecs.2019.07.05.
Abstract: Educational data mining (EDM) is an emerging interdisciplinary research area concerned with analyzing and studying data from academic databases to better understand the students and the educational settings. In most of the Asian countries, it is a challenging task to perform EDM due to the diverse characteristics of the educational data. In this study, we have performed students’ educational performance prediction, pattern analysis and proposed a generalized framework to perform rigorous educational analytics. To validate our proposed framework, we have also conducted extensive experiments on a real-world dataset that has been prepared by the transcript data of the students from the Marketing department of a renowned university in Bangladesh. We have applied six state-of-the-art classification algorithms on our dataset for the prediction task where the Random Forest model outperforms the other models with accuracy 94.1%. For pattern analysis, a tree diagram has been generated from the Decision Tree model.
Islam Rifat, Md Rifatul, Abdullah Al Imran, and A. S. M. Badrudduza. “EduNet: A Deep Neural Network Approach for Predicting CGPA of Undergraduate Students.” 2019 1st International Conference on Advances in Science, Engineering and Robotics Technology (ICASERT). IEEE, May 2019. https://doi.org/10.1109/icasert.2019.8934616.
Abstract: Educational Data Mining (EDM) is an emerging research field concerned with the application of data mining, machine learning, and statistics in the discipline of education. Many researchers have already focused on EDM and exploring the educational data using several traditional data mining techniques to improve the educational performance of the students by extracting the concealed patterns and predicting the final outcome. In this study, we aim to propose a Deep Neural Network (DNN) based model to predict the final CGPA of the undergraduate business students with a minimal error than the traditional approaches. We have considered the performance of a decision tree model as the baseline performance. Experiments in this study have shown that our proposed DNN model can predict the CGPA with a significantly minimal error rate. To measure the performance of our model we have considered the three evaluation metrics namely Mean Squared Error (=0.008), Mean Absolute Error (=0.067), and Mean Absolute Percentage Error (=2.074). Our proposed model has successfully shown a promising prediction performance by reducing the MSE, MAE, and MAPE by 0.0146, 0.0431, and 6.043 respectively, compared to the baseline model.
Abdullah Al Imran, Md Nur Amin, Md Rifatul Islam Rifat, and Shamprikta Mehreen. “Deep Neural Network Approach for Predicting the Productivity of Garment Employees.” 2019 6th International Conference on Control, Decision and Information Technologies (CoDIT). IEEE, April 2019. https://doi.org/10.1109/codit.2019.8820486.
Abstract: The garment industry is one of the most dominating industries in this era of industrial globalization. It is a highly labor-intensive industry that requires a large number of human resources to produce its goods and fill up the global demand for garment products. Because of the dependency on human labor, the production of a garment company comprehensively relies on the productivity of the employees who are working in different departments of the company. A common problem in this industry is that the actual productivity of the garment employees sometimes does not meet the targeted productivity that was set for them by the authorities to meet the production goals in due time. When the productivity gap occurs, the company faces a huge loss in production. This study aims to solve this problem by predicting the actual productivity of the employees. To achieve this aim, a Deep Neural Network (DNN) model has been proposed to predict the actual productivity of the employees. The experimental results of this study have shown that the proposed model yields a promising prediction performance with a minimal Mean Absolute Error (=0.086) which is less than the baseline performance error (=0.15). Such prediction performance can indisputably help the manufacturers to set an accurate target, minimize the production loss and maximize the profit.
Rafsunjani, Siam, Rifat Sultana Safa, Abdullah Al Imran, Shamsur Rahim, and Dip Nandi. “An Empirical Comparison of Missing Value Imputation Techniques on APS Failure Prediction.” International Journal of Information Technology and Computer Science. MECS Publisher, February 8, 2019. https://doi.org/10.5815/ijitcs.2019.02.03.
Abstract: The Air Pressure System (APS) is a type of function used in heavy vehicles to assist braking and gear changing. The APS failure dataset consists of the daily operational sensor data from failed Scania trucks. The dataset is crucial to the manufacturer as it allows to isolate components which caused the failure. However, missing values and imbalanced class problems are the two most challenging limitations of this dataset to predict the cause of the failure. The prediction results can be affected by the way of handling these missing values and imbalanced class problem. In this paper, we have examined and presented the impact of five different missing value imputation techniques namely: Expectation Maximization, Mean Imputation, Soft Impute, MICE, and Iterative SVD in producing significantly better results. We have also performed an empirical comparison of their performance by applying five different classifiers namely: Naive Bayes, KNN, SVM, Random Forest, and Gradient Boosted Tree on this highly imbalanced dataset. The primary aim of this study is to observe the impact of the mentioned missing value imputation techniques in the enhancement of the prediction results, performing an empirical comparison to figure out the best classification model and imputation technique. We found that the MICE imputation and the random under-sampling techniques are the highest influential techniques for improving the prediction performance and false negative rate.
Wahid, Zaman, A. K. M. Zaidi Satter, Abdullah Al Imran, and Touhid Bhuiyan. “Predicting Absenteeism at Work Using Tree-Based Learners.” Proceedings of the 3rd International Conference on Machine Learning and Soft Computing - ICMLSC 2019. ACM Press, 2019. https://doi.org/10.1145/3310986.3310994.
Abstract: Absenteeism at workplace acts as a crucial role in demonstrating the productive and profitable capacity of a company. Thus the knowledge of absenteeism of employees' becomes the foundation for an organization in its multiple dimensions. Because the proper determination of employees' profile allows the identification of excesses of occurrences of certain morbidities. The early absenteeism research primarily focused on predicting the characteristics and the categories of diseases of employees that make them perform higher absenteeism at workplace. However, predicting the absenteeism time of employees using different machine learning classifiers is able to give the researches a new dimension in line with the intention of revealing the underlying causes and patterns of absenteeism. In this paper, we have applied 4 prominent machine learning algorithms namely Decision Tree, Gradient Boosted Tree, Random Forest, and Tree Ensemble on the absenteeism dataset of a courier company in Brazil in order to predict the absenteeism time of employees at work as well as the best classifier. Based on the 7 evaluation metrics such as True Positive, True Negative, False Positive, False Negative, Sensitivity, Specificity, and Accuracy we found that Gradient Boosted Tree produced the best result with an accuracy rate of 82% whereas Tree Ensemble performed the lowest with the accuracy rate of 79%.
Abdullah Al Imran, Md Nur Amin, and Fatema Tuj Johora. “Classification of Chronic Kidney Disease Using Logistic Regression, Feedforward Neural Network and Wide & Deep Learning.” 2018 International Conference on Innovation in Engineering and Technology (ICIET). IEEE, December 2018. https://doi.org/10.1109/ciet.2018.8660844.
Abstract: Chronic kidney disease (CKD) is a global health burden that affects approximately 10% of the adult population in the world. It is also recognized as the top 20 causes of death worldwide. Unfortunately, there is no cure for CKD however, it is possible to slow down its progression and mollify the damage by early diagnosis of the disease. Due to a limited number of nephrologists, the early diagnosis of CKD is often not possible for most of the people. Therefore the use of modern computer-aided methods is necessary to aid the traditional CKD diagnosis system to be more efficient and accurate. In this research, our primary focus was to apply 3 modern machine learning techniques namely logistic regression, feedforward neural networks and wide & deep learning to diagnose CKD as well as finding the best performing technique by evaluating their diagnosis performance. To evaluate their performance, f1-score, precision, recall and AUC score was used for logistic regression and an additional loss score was considered for the feedforward neural networks and wide & deep model. We found the feedforward neural network as the best performing technique for CKD diagnosis with 0.99 f1-score, 0.97 precision, 0.99 recall and 0.99 AUC score. Logistic regression produced the lowest result among all and the wide & deep learning with a larger number of hidden layers and neurons found to be effective for larger datasets.
Abdullah Al Imran, Ananya Rahman, Humayoun Kabir, and Shamsur Rahim. “The Impact of Feature Selection Techniques on the Performance of Predicting Parkinson’s Disease.” International Journal of Information Technology and Computer Science. MECS Publisher, November 8, 2018. https://doi.org/10.5815/ijitcs.2018.11.02.
Abstract: Parkinson’s Disease (PD) is one of the leading causes of death around the world. However, there is no cure for this disease yet; only treatments after early diagnosis may help to relieve the symptoms. This study aims to analyze the impact of feature selection techniques on the performance of diagnosing PD by incorporating different data mining techniques. To accomplish this task, identifying the best feature selection approach was the primary focus. In this paper, the authors had applied five feature selection techniques namely: Gain Ratio, Kruskal-Wallis Test, Random Forest Variable Importance, RELIEF and Symmetrical Uncertainty along with four classification algorithms (K-Nearest Neighbor, Logistic Regression, Random forest, and Support Vector machine) on the PD dataset collected from the UCI Machine Learning repository. The result of this study was obtained by taking the four different subsets (Top 5, 10, 15, and 20 features) from each feature selection approach and applying the classifiers. The obtained result showed that in terms of accuracy, Random Forest Variable Importance, Gain Ratio, and Kruskal-Wallis Test techniques generated the highest 89% score. On the other hand, in terms of sensitivity, Gain Ratio and Kruskal-Walis Test approaches produced the highest 97% score. The findings of this research clearly indicated the impact of feature selection techniques on predicting PD and our applied methods outperformed the state-of-the-art performance.